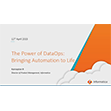
Big Data Fabrics Emerge to Ease Hadoop Pain
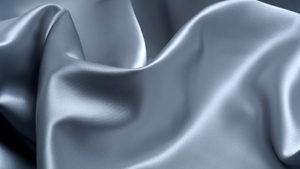
(photographyfirm/Shutterstock)
If Hadoop is leaving your data lake project all wet, you may be a good candidate for an emerging architectural concept called the big data fabric. As industry experts explain, big data fabrics bring a certain level of cohesion and automation to the processes and tools that companies are adopting as they try to get value out of big data.
Forrester analyst Noel Yuhanna explained the genesis of big data fabrics in his recent report on the matter. According to Yuhanna, the gap between the expectations that companies have with big data technologies like Hadoop and the real-world challenges of working with those technologies gave rise to big data fabrics.
Conceptually, a big data fabric is essentially a way of architecting a disparate collection of data tools that address key pain points in big data projects in a cohesive and self-service manner. Specifically, data fabric solutions deliver capabilities in the areas of data access, discovery, transformation, integration, security, governance, lineage, and orchestration challenges, according to Forrester.
Yuhanna writes: “The solution must be able to process and curate large amounts of structured, semi-structured, and unstructured data stored in big data platforms such as Apache Hadoop, MPP EDWs, NoSQL, Apache Spark, in-memory technologies, and other related commercial and open source platforms, including Apache projects. In addition, it must leverage big data technologies such as Spark, Hadoop, and in-memory as a compute and storage layer to assist the big data fabric with aggregation, transformation, and curation processing.”
Some data fabric vendors can tick off all of the capabilities in their products, while others tackle only a portion of the overall data fabric requirement. In any case, a common thread runs through all big data fabric solutions, in that they’re working toward a cohesive vision of data accessibility, while respecting the needs of automation, security, integration, and self-service.
Stitching Clouds into Fabric
The rise of cloud repositories also plays heavily into the emergence of big data fabrics, according to Ravi Shankar, chief marketing officer of Denodo Technologies, which was one of 11 big data fabric vendors profiled in Forrester’s recent report.
“The big data fabric is needed because, if you look at the underlying problem, before big data, data was divergent and located in many different systems,” Shankar tells Datanami. “Twenty years back, it was all on-premise. In the last 10 years, it has been evolving more to the cloud. Now it’s more into big data [platforms like Hadoop]. So data continues to be bifurcated across all these different points, and each of them adds some challenge.”
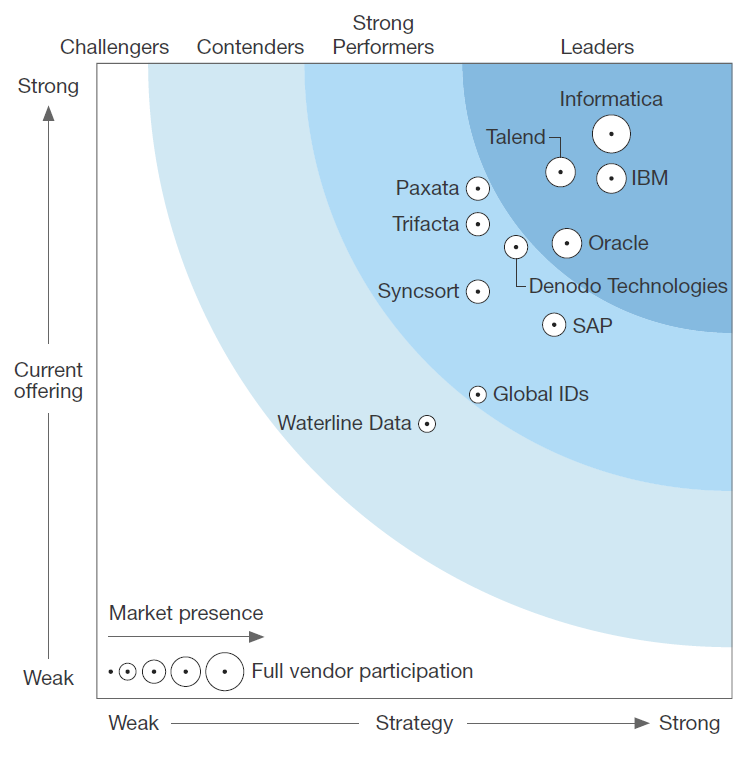
Source: The Forrester Wave: Big Data Fabric, Q4 2016 A Critical Platform For Enterprises To Succeed With Big Data Initiatives
The big data fabric concept is closely linked to other concepts gaining momentum in enterprise analytics, specifically the notion of virtual data lakes and logical data warehouses. While the original data lake theory held that all data would be loaded into Hadoop where it could be managed centrally, that largely has not happened. Instead, data continues to pile up in silos, including traditional analytic database and enterprise data warehouses (EDWs) like Teradata and Vertica, as well as more modern (but less structured) repositories like Hadoop, Spark, and NoSQL databases.
Shankar says data virtualization, which is Denodo’s specialty, is a key component of the big data fabric concept. “If you look at the vendors featured in [Forrester’s report] data virtualization is a key capability for companies like Informatica, IBM, and Oracle. They’re the ones who all have some sort of data virtualization capability to stitch the data all together from a fabric perspective and provide it for consumption.”
The current leaders of the emerging big data fabric market, according to Forrester’s report, are established giants in the data integration business: Informatica, IBM (NYSE: IBM), and Oracle (NYSE: ORCL). The other big data fabric in the leaders sector is Talend (NASDAQ: TLND), which has built a solid big data reputation on the back of open source ETL technology.
Companies that need to load data into Hadoop can do so with Talend’s ETL software, says Talend product marketing manager Ray Christopher. “But then you have more challenges around managing it and using it, and that’s where you need more mature capabilities like data governance,” he says.
“Or you might be moving from batch to real-time, or you might do machine learning or self-service,” he continues. “That, in a nutshell, is what the fabric does. It allows you to solve all your integration needs. It’s laser focused on helping you address the big challenges encountered in integrating big data in the cloud.”
Talend actually has a product called Talend Data Fabric that’s a superset of all of the software company’s capabilities, Christopher says. This product, which Talend updated last week with its Winter ’17 release, includes capabilities in the areas of data access, data cleansing and preparation, and data stewardship and governance, in addition to the core ETL.
Filling In Data Swamps
In some ways, the data fabric concept is a direct result of the poor results that many organizations have had with data lakes, most of which probably run on Hadoop, but may be running on other platforms, such as object storage systems.
The original data lake concept—where raw data is written into HDFS and processed or transformed only when it’s needed, or schema-on-read–is prone to result in a data swamp. Industry analysts at Gartner recently predicted that “Through 2018, 90 percent of deployed data lakes will become useless as they are overwhelmed with information assets captured for uncertain use cases.”
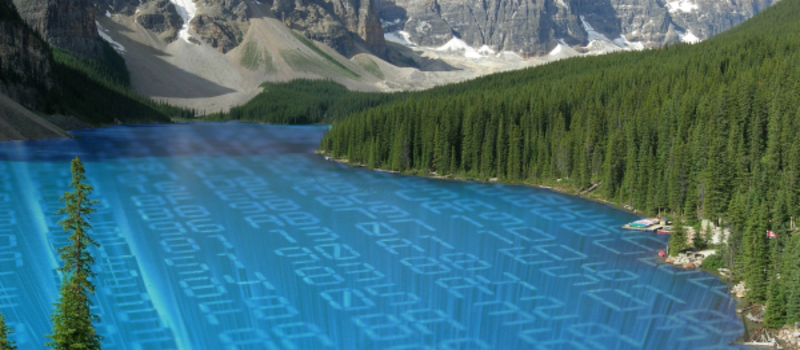
Data lakes are suffering from lack of automation, security, and governance (cherezoff/Shutterstock)
“Everyone is building data lakes,” Talend’s Christopher says. “We talk to prospects, and they say ‘I’m building a data lake. I went and bought a Hadoop distro, and my project is about to fail. The challenge is these vast data stores aren’t trustworthy. There are data quality issues. There’s lax governance, and security and privacy issues. And it just lacks that ubiquity. Oftentimes, the people who put the data in there are the only people who use it.”
Big data fabrics could be the savior of the data lake concept, as they unite various data repositories and provides common ways for users to access data processing tools. Nobody wants the IT department to be the driving force setting the requirements in big data projects. Getting buy-in from users in the departments that will actually be working with the data and the tools is critical. This is another reason why the big data fabric concept has legs: it’s more inclusive.
Today, there is a greater need to work with data than ever before. That core requirement is not changing. But the tools that we use to make sense of data are changing quickly. Hadoop and Spark will play important roles in future big data projects, as the storage and processing engines, respectively. But surrounding these key players will be an ensemble cast of tools and technologies needed to ensure that the data itself is treated in a consistent manner. The data fabric concept appears to be one way of delivering the tools that enable insights to be mined from data, consistently, securely, and in a governed and self-service manner.
Related Items:
Data Lake Showdown: Object Store or HDFS?
V is for Big Data Virtualization