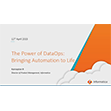
8 Best Practices for Approaching Master Data Governance in the Cloud
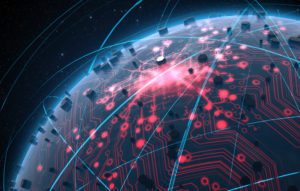
(Inked Pixels/Shutterstock)
Expanding your footprint to the cloud has gotten easier in recent years, and as a result, a growing community of data consumers are now motivated and energized to collect, capture, store, and analyze data for insights and business decision making. While more enterprise organizations are looking to go all-in on cloud, CIOs will learn that the nature of their data may require a hybrid approach to truly modernize their IT infrastructure. In fact, Gartner analysts believe hybrid IT will be the standard in 2019.
Despite traction, information management stakeholders still have concerns about the potential risk of managing their data, both in the cloud and on premises, for various reasons.
Enterprises have consistently cited security and governance as barriers to cloud adoption, looking for increased control where the cloud infrastructure is shared with a third party. There’s also a growing set of regulations that mandate stricter governance of personal data, such as the California Consumer Privacy Act (CCPA), the European Union’s General Data Protection Regulation (GDPR), and more. Not to mention democratization and data awareness. Moving data into any kind of data lake (cloud-based or on-premises) runs the risk of losing track of which data assets have been moved, the characteristics of their content, and details about their metadata. So discoverability becomes very important.
These factors highlight the need for increased data classification, cataloging, access control, data quality management, and monitoring of the flow of data through the organization (data lineage) as core data governance competencies that every organization should look for in a cloud provider. Addressing these risks, without abandoning cloud computing’s many benefits, makes it more important than ever to understand data governance in the cloud. And of course, know which parts are most critical.
Simply put, data governance encompasses the ways that people, processes, and technology can work together to enable business efficiency along with defined and agreed-upon data policies that ensure compliance is met. As a given company generates more data and moves it to the cloud, they need to consider a comprehensive approach to data governance. Why? Because good data governance can inspire customer trust, allow the company to extract more value from their data and therefore lead to more competitive offerings and improvements in, for example, customer experience.
There are well-known frameworks out there that can be great resources for how to approach data governance (i.e. Gartner framework , Forrester framework ). But these steps aren’t always linear or the same for every organization.
With that in mind, here are eight best practices for any company to consider as they approach data governance in the cloud:
1. Data Discovery and Assessment
Cloud-based environments offer an economical option for creating and managing data lakes, but the risk remains for ungoverned migration of data assets. You can mitigate this with improved data discovery and assessment processes. By identifying data assets within a primary, hybrid, or multi-cloud environment you can trace and record each data asset by origin, lineage, object metadata, and discovery what transformations have been applied to the data. Better data discovery means that users can find the data they need, when they need it, which leads to efficiency and better, data-driven decision making.
2. Data Classification and Organization
Properly evaluating a data asset and scanning the content of its different attributes can help categorize the data asset for subsequent organization. This process can also infer whether the object contains sensitive data, and if so, classifying each data asset in terms of the different levels of data sensitivity, including personal and private data, confidential data, and intellectual property. To implement data governance in the cloud, you’ll need to profile and classify sensitive data in order to inform which governance policies and procedures apply to the data.
3. Data Cataloging and Metadata Management
Once your data assets are assessed and classified, it is crucial that you document your learnings so that your communities of data consumers have visibility into your organization’s data landscape. You need to maintain a data catalog that contains structural metadata, data object metadata, and the assessment of levels of sensitivity in relation to the governance directives (such as compliance with one or more data privacy regulations). The data catalog not only allows data consumers to view this information, but it can also serve as part of a reverse index for search and discovery, both by phrase and (given the right ontologies) by concept. It is also important to understand the format of structured and semi-structured data objects, and allow your systems to handle these data types differently, as necessary.
4. Data Quality Management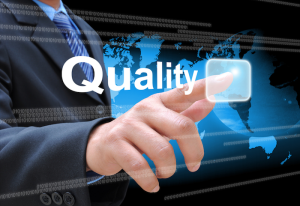
Different data consumers may have different data quality requirements, so it’s important to provide a means to document data quality expectations, as well as techniques and tools for supporting the data validation and monitoring process. These management processes include creating controls for validation, enabling quality monitoring and reporting, supporting the triage process for assessing the level of incident severity, enabling root cause analysis and recommendation of remedies to data issues, and data incident tracking. The right processes for data quality management will provide measurably trustworthy data for analysis.
5. Data Access Management
There are two aspects of governance for data access. The first aspect is the provisioning of access to available assets. It’s important to provide data services that allow data consumers to access their data, and fortunately, most cloud platform providers provide methods for developing data services. The second aspect is prevention of improper or unauthorized access. It’s important to define identities, groups, and roles, and assign access rights to establish a level of managed access. This best practice involves managing access services as well as interoperating with the cloud provider’s identity and access management (IAM) services by defining roles, specifying access rights, and managing and allocating access keys for ensuring that only authorized and authenticated individuals and systems are able to access data assets according to defined rules.
6. Auditing
Organizations must be able to assess their systems to make sure that they are working as designed. Monitoring, auditing and tracking (who did what and when and with what information) helps security teams gather data, identify threats, and act on them before they result in business damage or loss. It’s important to perform regular audits: check the effectiveness of controls in order to quickly mitigate threats and evaluate overall security health.
7. Data Protection
Perimeter security is not, and never has been sufficient for protecting sensitive data. Attempting to prevent someone from breaking into your system has limited success, but at some point, your data may become exposed. It’s important to institute additional methods of data protection to ensure that exposed data cannot be read, including encryption at rest, encryption in transit, data masking, and permanent deletion.
8. Data Literacy
A critical component to an organization’s success with data governance relies on education, training, and a true understanding of what can and can’t be done with your data. Technology on its own isn’t enough – it takes people, process, policies to drive organizational change and enable users to see and protect the value of their data as a business asset.
Overall, organizations will likely reap immense benefits as they promote a data-driven culture – a large part of which involves a strong understanding of data governance. By putting these recommendations into practice, information management professionals will be one step closer.
About the author: Evren Eryurek, PhD is the leader of Data Analytics and Data Management portfolio of Google Cloud. As the director of product management, Evren’s responsibilities cover Streaming Analytics, Dataflow, Beam, Messaging (Pub/Sub & Confluent Kafka), Data Governance, Data Catalog & Discovery and Data Marketplace as the Director of Product Management. Prior to joining Google, he was the SVP & Software Chief Technology Officer for GE Healthcare, near $20 billion segments of GE. A graduate of the University of Tennessee, Evren holds a master’s and a doctorate degree in Nuclear Engineering and over 60 U.S. patents.
Related Items:
Why Investing in Your Team’s Data Culture Could Be the Most Important Money You Spend
The State of Storage: Cloud, IoT, and Data Center Trends
February 21, 2025
- Kioxia and Sandisk Unveil Next-Gen 3D Flash Memory Tech Achieving 4.8Gb/s NAND Interface Speed
- DataChat’s Gen AI Platform for Analytics on Track for Continued Growth in 2025
- Lucidworks Launches AI-Powered Commerce Studio and Analytics Studio, Available in New Fast-Start Packages
- Arize AI Secures $70M Series C to Expand AI Observability and LLM Evaluation
February 20, 2025
- Accenture Invests in Voltron Data to Help Organizations Use GPU Tech to Simplify Large-Scale Data Processing
- DDN Unveils Infinia 2.0 to Streamline AI Data Management and GPU Utilization
- Together AI Raises $305M Series B to Power AI Model Training and Inference
- Starburst Closes Record FY25, Fueled by Rising AI Demand and Growing Enterprise Momentum
- GridGain Brings Apache Ignite Community Together for Ignite Summit 2025
- Elasticsearch Open Inference API now Supports Jina AI Embeddings and Rerank Model
- HarperDB Named an IDC Innovator for Edge Inference Delivery
- EDB Postgres AI Outperforms Oracle, SQL Server, and MongoDB in New Benchmark
- CData and Ellie.ai Partner to Streamline Enterprise Data Modeling
- Privacera Aligns AI Governance with NIST Standards to Mitigate AI Risks
February 19, 2025
- AtScale and Snowflake Announce Integration with Cortex Analyst to Deliver Trustworthy Natural Language Queries
- Sawmills Exits Stealth with $10M to Tackle Skyrocketing Observability Costs Using AI
- Prophecy Finds GenAI Boosting Data Team Productivity by Up to 50%
- CTERA Enhances Cybersecurity and AI Capabilities in Record-Setting Year
- VAST Data Introduces Event Broker for AI-Driven Real-Time Streaming
- DiffusionData Announces Free Trial of Diffusion Cloud
- OpenTelemetry Is Too Complicated, VictoriaMetrics Says
- What Are Reasoning Models and Why You Should Care
- Three Ways Data Products Empower Internal Users
- Keeping Data Private and Secure with Agentic AI
- Memgraph Bolsters AI Development with GraphRAG Support
- Three Data Challenges Leaders Need To Overcome to Successfully Implement AI
- PayPal Feeds the DL Beast with Huge Vault of Fraud Data
- What Leonardo DaVinci Teaches Us About Data Management
- Demystifying AI: What Every Business Leader Needs to Know
- Top-Down or Bottom-Up Data Model Design: Which is Best?
- More Features…
- Meet MATA, an AI Research Assistant for Scientific Data
- AI Agent Claims 80% Reduction in Time to Complete Data Tasks
- DataRobot Expands AI Capabilities with Agnostiq Acquisition
- Snowflake Unleashes AI Agents to Unlock Enterprise Data
- EDB Says It Tops Oracle, Other Databases in Benchmarks
- Collibra Bolsters Position in Fast-Moving AI Governance Field
- Microsoft Open Sources Code Behind PostgreSQL-Based MongoDB Clone
- AI Making Data Analyst Job More Strategic, Alteryx Says
- VAST Data Expands Platform With Block Storage And Real-Time Event Streaming
- Databricks Unveils LakeFlow: A Unified and Intelligent Tool for Data Engineering
- More News In Brief…
- Informatica Reveals Surge in GenAI Investments as Nearly All Data Leaders Race Ahead
- Gartner Predicts 40% of Generative AI Solutions Will Be Multimodal By 2027
- PEAK:AIO Powers AI Data for University of Strathclyde’s MediForge Hub
- DataRobot Acquires Agnostiq to Accelerate Agentic AI Application Development
- Cloudera Welcomes Tom Brady as Keynote Speaker at ELEVATE26
- Starburst Closes Record FY25, Fueled by Rising AI Demand and Growing Enterprise Momentum
- TigerGraph Launches Savanna Cloud Platform to Scale Graph Analytics for AI
- EY and Microsoft Unveil AI Skills Passport to Bridge Workforce AI Training Gap
- Alluxio Enhances Enterprise AI with Version 3.5 for Faster Model Training
- DeepSeek-R1 models now available on AWS
- More This Just In…