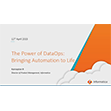
Selecting a Data Lake ETL Platform? Here Are 6 Questions to Ask
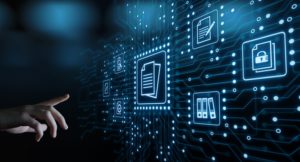
(Alexander Supertramp/Shutterstock)
Not all data lakes are created equal. If your organization wants to adopt a data lake solution to simplify and more easily operate your IT infrastructure and store enormous quantities of data without requiring extended data transformation, then go for it.
But before you do, understand that simply dumping all your data into object storage such as AWS S3 doesn’t exactly mean you will have a working data lake.
The ability to use that data in analytics or machine learning requires converting that raw information into organized datasets you can use for SQL queries, and this can only be done via extract-transform-load (ETL) flows.
Data lake ETL platforms are available in a full range of options – from open-source to managed solutions to custom-built. Whichever tool you select, it’s important to differentiate data lake ETL challenges from traditional database ELT demands – and seek the platform that overcomes these obstacles.
Ask yourself which ETL solution:
1. Effectively Conducts Stateful Transformations
Traditional ETL frameworks allow for stateful operations like joins and aggregations to enable analysts to work with data from multiple sources; this is difficult to implement with a decoupled architecture.
Stateful transformations can occur by relying on extract-load-transform (ELT) – i.e., sending data to an “intermediary” database and using the database’s SQL, processing power and already amassed historical data. After transformation, the information is loaded into the data warehouse tables.
Data lakes, aiming to reduce cost and complexity by avoiding decoupled architecture, cannot depend on databases for every activity. You’ll need to look for an ETL tool that can conduct stateful transformations in-memory and needs no additional database to sustain joins and aggregations.
2 Extracts Schema from Raw Data
Organizations customarily use data lakes as a storehouse for raw data in a structured or semi-structured arrangement vs databases, which are predicated on structured tables. This poses numerous challenges.
One, in order to query data, can the data lake ETL tool draw out a schema (without which querying is not possible) from the raw data – and bring it up to date as changes in data and data structure come about? And two — this is an ongoing struggle — can the ETL tool effectively make queries with nested data?
3 Improves Query Performance Via Optimized Object Storage
Have you tried to read raw data straight from a data lake? Unlike using a database’s optimized file system that quickly sends back query results, doing the same operation with a data lake can be quite frustrating performance-wise.
To get optimal results, your ETL framework should continually store data in columnar formats and merge small files to the 200mb-1gb range. Unlike traditional ELT tools that only need to write the data once to its target database, data lake ETL should support the ability to write multiple copies of the same data based on the queries you will want to run and the various optimizations required for your query engines to be performant.
4 Easily Integrates with the Metadata Catalog
You’ve chosen the data lake approach for its flexibility — store large quantities if data now but analyze it later — and the ability to handle a wide range of analytics use cases. Such an open architecture should keep metadata separate from the engine that queries it, so you can easily change these query engines or use several simultaneously for the same data.
This means the data lake tool you select should reinforce this open architecture, i.e., be seamlessly merged with the metadata catalog. This allows the metadata to be easily “queryable” by various services because it is both stored in the catalog and still dovetails with every adjustment in schema, partition, and location of objects.
5 Replays Historical Data
Say you wanted to test a hypothesis by looking at stored data on a historical basis. This is difficult to accomplish with the traditional database option, where data is stored in a mutable condition, and in which running such a query could be prohibitive in terms of cost, stress, and tension between operations and analysis.
It’s easy to do with a data lake. In data lakes, stored raw data remains continuously available – it only transformed after extraction. Therefore, having a data lake allows you got “travel back in time,” seeing the exact state of the data as it was collected.
“Traditional” databases don’t allow for that, as the data is only stored in its transformed state.
6 Updates Tables Periodically
Data lakes, unlike databases that allow you to update and make deletions to tables, contain partitioned files that enable an append or add-only feature. If you want to store transactional data, implement change data capture in the data lake, or delete particular data for GDPR compliance, you’ll have difficulty doing so.
Make sure that the data lake ETL tools you choose have the ability to sidestep this obstacle. Your solution should be able to allow upserts, a system that lets you insert new records or update existing ones, in the storage layer and in the output tables.
About the author: Ori Rafael is the CEO and co-founder of Upsolver, a provider of a self-service data lake ETL platform that bridges the gap between data lakes and data consumers. Ori has worked in IT for nearly two decades and has an MBA from Tel Aviv University.
Related Items:
Merging Batch and Stream Processing in a Post Lambda World
March 31, 2025
- Tamr Reports 65% ARR Growth, Expands Customer Base and Executive Team
- Fujitsu Partners with Macquarie University to Launch Online AutoML Course
March 28, 2025
- Lockheed Martin and Google Cloud Partner to Advance GenAI for National Security
- Datadobi Releases StorageMAP 7.2 with Enhanced Metadata and Object Storage Discovery
- Carnegie Mellon Expands AI Research with Google-Powered Cloud GPU Cluster
- Trillion Parameter Consortium Partners with Tabor Communications to Launch Global AI for Science Conference, TPC25
March 27, 2025
- IBM Expands On-Prem Offerings with Storage Ceph as a Service
- Dataminr Partners with WWT to Launch Unified Cyber-Physical Threat Intelligence Platform
- Dataiku Announces 2025 Partner Award Winners
- Marvell Showcases PCIe Gen 6 Optical Interconnect for AI Infrastructure
- Akamai Launches Cloud Inference to Boost AI Workloads at the Edge
- Prophecy Introduces Fully Governed Self-Service Data Preparation for Databricks SQL
- Verdantis Launches Next-Gen AI Solutions to Transform Enterprise Master Data Management
- TDengine Releases TDgpt, Extending the Power of AI to the Industrial Sector
March 26, 2025
- Quest Adds GenAI to Toad to Bridge the Skills Gap in Modern Database Management
- SymphonyAI Expands Industrial AI to the Edge with Microsoft Azure IoT Operations
- New Relic Report Reveals Media and Entertainment Sector Looks to Observability to Drive Adoption of AI
- Databricks and Anthropic Sign Deal to Bring Claude Models to Data Intelligence Platform
- Red Hat Boosts Enterprise AI Across the Hybrid Cloud with Red Hat AI
March 25, 2025
- PayPal Feeds the DL Beast with Huge Vault of Fraud Data
- OpenTelemetry Is Too Complicated, VictoriaMetrics Says
- Accelerating Agentic AI Productivity with Enterprise Frameworks
- When Will Large Vision Models Have Their ChatGPT Moment?
- Your Next Big Job in Tech: AI Engineer
- Data Warehousing for the (AI) Win
- Nvidia Touts Next Generation GPU Superchip and New Photonic Switches
- Alation Aims to Automate Data Management Drudgery with AI
- Can You Afford to Run Agentic AI in the Cloud?
- What Benchmarks Say About Agentic AI’s Coding Potential
- More Features…
- Clickhouse Acquires HyperDX To Advance Open-Source Observability
- NVIDIA GTC 2025: What to Expect From the Ultimate AI Event?
- Grafana’s Annual Report Uncovers Key Insights into the Future of Observability
- Google Launches Data Science Agent for Colab
- IBM to Buy DataStax for Database, GenAI Capabilities
- EDB Says It Tops Oracle, Other Databases in Benchmarks
- Databricks Unveils LakeFlow: A Unified and Intelligent Tool for Data Engineering
- Reporter’s Notebook: AI Hype and Glory at Nvidia GTC 2025
- Weaviate Introduces New Agents to Simplify Complex Data Workflows
- Mathematica Helps Crack Zodiac Killer’s Code
- More News In Brief…
- Gartner Predicts 40% of Generative AI Solutions Will Be Multimodal By 2027
- Snowflake Ventures Invests in Anomalo for Advanced Data Quality Monitoring in the AI Data Cloud
- Seagate Unveils IronWolf Pro 24TB Hard Drive for SMBs and Enterprises
- NVIDIA Unveils AI Data Platform for Accelerated AI Query Workloads in Enterprise Storage
- Accenture Invests in OPAQUE to Advance Confidential AI and Data Solutions
- Alation Introduces Agentic Platform to Automate Data Management and Governance
- Gartner Identifies Top Trends in Data and Analytics for 2025
- Qlik Survey Finds AI at Risk as Poor Data Quality Undermines Investments
- Palantir and Databricks Announce Strategic Product Partnership to Deliver Secure and Efficient AI to Customers
- HighByte Launches API Builder for Industrial Data
- More This Just In…