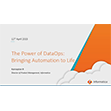
The Cure for Kubernetes Storage Headaches: Break Your Data Free
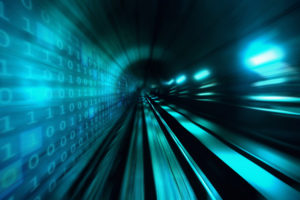
(Khing Choy/Shutterstock)
If you’re using Kubernetes, there’s likely a simple reason why: Because it makes your life easier. That is, after all, the whole premise behind container-based orchestration. Infrastructure becomes disposable. Spin it up when you need it, throw it away when you’re done, and let Kubernetes worry about the underlying infrastructure, so you don’t have to think too much about it.
At least, that’s how things are supposed to work. As you know if you’ve actually set up workloads that depend on persistent data, there’s one big asterisk – storage.
As great as Kubernetes is at abstracting away compute and networking infrastructure, it just doesn’t work that way for storage when your apps are stateful and data is persistent. Your application still must know all about the underlying storage infrastructure to find its way to the data you need. And not just the location of that data, but all the other fine-grained considerations (performance, protection, resiliency, data governance, and cost) that come with different kinds of storage infrastructure, that most data scientists don’t want to think about.
Why, in a cloud-native world where we’ve automated away the management of so much underlying hardware complexity, is storage still so painful? Two words: data silos.
As long as we continue to manage data via the different infrastructures it lives on, rather than focusing on the data itself, we’ll inevitably end up juggling islands of storage, with all the headaches that come with them. Fortunately, this is not an intractable problem. By changing the way we think about data management, from an infrastructure-centric to a data-centric approach, we can use Kubernetes to give us what was promised in the first place: making storage SEP (Someone Else’s Problem).
Virtualize Your Data
When the data you need is sprawled across different storage silos, each with its own unique attributes (this-or-that cloud, on-premises, object, high-performance, etc.), there’s just no way to abstract away infrastructure considerations. Someone still has to answer all those questions about performance and cost and data governance to set up your pipeline. (And if that person is an IT admin you call for help, you can bet they cringe every time your name pops up on a ticket. Because they know they’re going to be spending the day wrestling with arcane infrastructure interfaces to wrangle your data across all the different copies and data stores, and there’s no way they’re getting that done before lunch.)
The only way to get rid of that headache—the only way to actually realize the speed and simplicity that Kubernetes is supposed to give you—is by virtualizing your data. Basically, you need an intelligent abstraction layer between your data and all your diverse storage infrastructure. That abstraction layer should let see and access your data everywhere, without having to worry about whether a given infrastructure has the right cost, location, or governance for what you’re doing, and without having to constantly make new copies.
Making this happen is not as difficult as it sounds. The key: metadata. When you can encode all the data requirements, context, or lineage considerations into metadata that follows your data everywhere, then it no longer matters which infrastructure data happens to reside on at any given moment. Now, when you’re setting up a data pipeline, you can work entirely with metadata. And your virtualization layer can use AI/ML to automatically handle all the underlying data management and infrastructure considerations for you.
Capitalize on Infrastructure Abstraction
Once you have your virtualization layer in place, and you’re handing data management via metadata, you can do all sorts of things you couldn’t do before. Things like:
- Eliminate data silos: Now, it doesn’t matter which infrastructure the data you need lives on or where that infrastructure is located. To your application, all those previously siloed storage resources (on-premises, cloud, hybrid, archival) just look like a universal global namespace.
- Access storage resources programmatically: Since you’re dealing in metadata—instead of a dozen different underlying hardware infrastructures—you can now set up your pipeline and access your data via declarative statements: I need this data, with this performance, and that’s all I really care about. The intelligent virtualization layer then goes and makes it happen, without your application (or your overburdened IT admin) needing to tell it exactly how.
- Make data management self-service: Data scientists don’t want to worry about comparing the costs of different storage types, enabling data protection, or making sure they’re meeting security and compliance requirements every time they set up a pipeline. (For that matter, your IT and security teams likely don’t want data scientists making those choices either—unless they like having everything run on the most expensive storage, without proper compliance.) Once you separate management of metadata from data, that all goes away. Storage administrators can set guardrails by configuring basic policy once. Users can then self-service most of their data management needs from then on—without opening a ticket, and without the errors that arise when they’re manually making those calls every time they set up a pipeline.
- Continually enrich your data: When your system supports customizable, extensible metadata, you can now do all sorts of interesting things. For example, you can build recursive processes, where you run data through a system, get some results, add those results back to the metadata, and run the job again. You can begin to build deep contextual understanding of the data around the data. The more that data is processed and used, the richer it becomes for other jobs in the future. And, that intelligence now always lives with that data everywhere, for any other application or data scientist who wants to use it. It’s not restricted to one copy, on one island of storage hidden away somewhere.
Unshackle Your Data
All of these things are possible when you virtualize your data, just because metadata is so much more flexible to work with than siloed storage infrastructures. The storage considerations that used to come with setting up and orchestrating your data pipeline can now just happen for you. Your storage resources become programmable, self-service, and automatically compliant, typically requiring no manual intervention.
All of a sudden, you’re actually living the reality that Kubernetes and software-defined storage was always supposed to deliver. Storage is software-defined, programmable, and consistent across hybrid cloud environments, regardless of the underlying infrastructure. Your data is richer and more flexible. Your IT team no longer keeps a blown-up picture from your ID card on the wall to throw darts at. Most important, you’re spending a lot more of your time actually working with your data—instead of worrying about where it lives.
About the author: Hammerspace Vice President of Product Marketing Brendan Wolfe has a long history of product marketing and product management in enterprise IT from servers to storage. Working with both large companies and startups, Brendan helps bring innovative products to new emerging markets.
Related Items:
The State of Storage: Cloud, IoT, and Data Center Trends
Blurred Storage Lines: Clouds That Appear Like On-Prem
April 24, 2025
- StorONE and Phison Unveil Storage Platform Designed for LLM Training and AI Workflows
- Dataminr Raises $100M to Accelerate Global Push for Real-Time AI Intelligence
- Elastic Announces General Availability of Elastic Cloud Serverless on Google Cloud Marketplace
- CNCF Announces Schedule for OpenTelemetry Community Day
- Thoughtworks Signs Global Strategic Collaboration Agreement with AWS
April 23, 2025
- Metomic Introduces AI Data Protection Solution Amid Rising Concerns Over Sensitive Data Exposure in AI Tools
- Astronomer Unveils Apache Airflow 3 to Power AI and Real-Time Data Workflows
- CNCF Announces OpenObservabilityCon North America
- Domino Wins $16.5M DOD Award to Power Navy AI Infrastructure for Mine Detection
- Endor Labs Raises $93M to Expand AI-Powered AppSec Platform
- Ocient Announces Close of Series B Extension Financing to Accelerate Solutions for Complex Data and AI Workloads
April 22, 2025
- O’Reilly Launches AI Codecon, New Virtual Conference Series on the Future of AI-Enabled Development
- Qlik Powers Alpha Auto Group’s Global Growth with Automotive-Focused Analytics
- Docker Extends AI Momentum with MCP Tools Built for Developers
- John Snow Labs Unveils End-to-End HCC Coding Solution at Healthcare NLP Summit
- PingCAP Expands TiDB with Vector Search, Multi-Cloud Support for AI Workloads
- Qumulo Launches New Pricing in AWS Marketplace
April 21, 2025
- PayPal Feeds the DL Beast with Huge Vault of Fraud Data
- OpenTelemetry Is Too Complicated, VictoriaMetrics Says
- Will Model Context Protocol (MCP) Become the Standard for Agentic AI?
- Thriving in the Second Wave of Big Data Modernization
- What Benchmarks Say About Agentic AI’s Coding Potential
- Google Cloud Preps for Agentic AI Era with ‘Ironwood’ TPU, New Models and Software
- Google Cloud Fleshes Out its Databases at Next 2025, with an Eye to AI
- Can We Learn to Live with AI Hallucinations?
- Monte Carlo Brings AI Agents Into the Data Observability Fold
- AI Today and Tomorrow Series #3: HPC and AI—When Worlds Converge/Collide
- More Features…
- Grafana’s Annual Report Uncovers Key Insights into the Future of Observability
- Google Cloud Cranks Up the Analytics at Next 2025
- New Intel CEO Lip-Bu Tan Promises Return to Engineering Innovation in Major Address
- AI One Emerges from Stealth to “End the Data Lake Era”
- SnapLogic Connects the Dots Between Agents, APIs, and Work AI
- Snowflake Bolsters Support for Apache Iceberg Tables
- GigaOM Report Highlights Top Performers in Unstructured Data Management for 2025
- Supabase’s $200M Raise Signals Big Ambitions
- New Benchmark for Real-Time Analytics Released by Timescale
- Big Data Career Notes for March 2025
- More News In Brief…
- Gartner Predicts 40% of Generative AI Solutions Will Be Multimodal By 2027
- MinIO: Introducing Model Context Protocol Server for MinIO AIStor
- Dataiku Achieves AWS Generative AI Competency
- AMD Powers New Google Cloud C4D and H4D VMs with 5th Gen EPYC CPUs
- Prophecy Introduces Fully Governed Self-Service Data Preparation for Databricks SQL
- Seagate Unveils IronWolf Pro 24TB Hard Drive for SMBs and Enterprises
- CData Launches Microsoft Fabric Integration Accelerator
- MLCommons Releases New MLPerf Inference v5.0 Benchmark Results
- Opsera Raises $20M to Expand AI-Driven DevOps Platform
- GitLab Announces the General Availability of GitLab Duo with Amazon Q
- More This Just In…