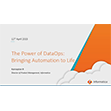
Actionable Strategies for Mitigating Risks & Driving Adoption with Responsible Machine Learning
Like other powerful technologies, AI and machine learning (ML) present significant opportunities. To reap the full benefits of Machine Learning (ML), organizations must also mitigate the considerable risks it presents. In order to drive deeper insights, address privacy and security vulnerabilities, and prevent the perpetuation of historical human or data bias, organizations should consider how core frameworks for responsible AI / ML enable the adoption of AI while accounting for its known risks.
What is Responsible AI / ML?
Explainable AI and the pursuit of using technology and statistical methods to explain Machine Learning models, quickly became a much larger question. Best practices in applying AI is not just a statistical question, but a people and process question as well, which forms the key elements of Responsible AI / ML. In order to achieve maximum transparency and understanding of AI, it is imperative to address and understand the full view of models and their impact. There are six categories that comprise the most critical themes in Responsible AI / ML: Explainable AI, Interpretable Machine Learning technology, Ethical AI, Secure AI, Human-Centered AI, and Compliance.
How to Innovate Responsibly?
In their new O’Reilly report “Responsible Machine Learning” authors Patrick Hall, Navdeep Gill, and Ben Cox focus on the technical issues of ML as well as human-centered issues such as security, fairness, and privacy. The goal is to promote human safety in ML practices so that in the near future, there will be no need to differentiate between the general practice and the responsible practice of ML. This report explores:
- People: Humans in the Loop — Why an organization’s ML culture is an important aspect of responsible ML practice
- Processes: Taming the Wild West of Machine Learning Workflows — Suggestions for changing or updating your processes to govern ML assets
- Technology: Engineering ML for Human Trust and Understanding — Tools that can help organizations build human trust and understanding into their ML systems
- Actionable Responsible ML Guidance — Core considerations for companies that want to drive value from ML
Download the report today to learn a set of actionable best practices for people, processes, and technology that can enable organizations to innovate with ML in a responsible manner.