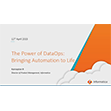
Advanced Analytics and AI: Two Divergent But Synergistic Capabilities
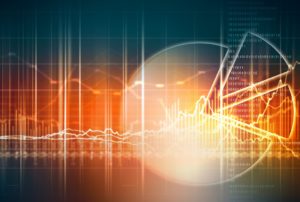
(Sergey-Nivens/Shutterstock)
Two commonly cited use cases for advanced analytics across financial services entail risk management and fraud detection; specifically, the use of advanced analytics to detect and reduce incidents of false positives.
Many financial services institutions (FSIs) are still working on optimizing these solutions and contrary to what some may believe, artificial intelligence has not rendered advanced analytics obsolete. In fact, many robust AI solutions rely on insights weaned through advanced analytics, and those organizations that are not yet ready to hand off their reins entirely to AI may find solace in mastering advanced analytics first.
Another very popular use of advanced analytics has been in the field of retail: advertisement fraud detection involves the use of analytics to detect successful campaigns and avoid wasteful spending. FSIs are perfect candidates for deploying similar advanced analytics software for ad fraud detection. For instance, in a mock home loan (or refinancing of a vehicle) use case, they can be used to detect if:
- A home loan campaign found success with the target
- Was the audience more receptive (if at all), at certain times of the day or at a certain time in the year?
- Should such a campaign only target customers who expressed their interest in securing a loan or had queries around a mortgage?
- Did in-person query result in higher issue resolution rate, or did customers report better service quality when interacting with bots?
Advanced analytics can uncover answers to these questions and can be used to devise prescriptive solutions. However, exploration need not end there. By bringing AI into the mix, specifically machine learning, FSIs can uncover parallel revenue streams, simply because machine learning is unpresumptuous.
Why Do Subtleties Between AI and Advanced Analytics Matter?
In a standard machine learning model, first, a question is posed, then data is gathered that may be perceived as a means to uncovering a plausible solution. That data is then analyzed and an algorithm or model is created. The model is tested and feedback from different stakeholders is gathered to evaluate the model’s ability to address real-world problems. That feedback is then fed back into the model and the loop begins again, until a desired accuracy level is achieved. Therefore, limitations of an existing advanced analytics solution, which tends to be static or fixed, can be overcome with a complimentary, dynamic AI solution.
Advanced analytics are a class of sophisticated algorithms but due to their static nature do not consider future rolling data. Inevitably, they can become obsolete over time. Going back to the home loan problem, a senior level executive might associate drop in home loan applications in a given quarter with a slowing economy, slow wage growth, an aging population or one that’s too young, or unrealistic real estate prices. These are just some of the conceivable factors that a human programmer might use to create an advanced analytics solution.
However, it is possible for a machine learning model to uncover entirely new, seemingly unrelated (at least to a human programmer) insights. For example, the mass exodus of three multinational corporations from a local community would mean many of their workers also left the community. Consequently, more homes were available than there were buyers, resulting in a price drop. Or perhaps a new regulation is on the horizon that would greatly incentivize new homeowners, consequently fewer people are now wanting to rent as they would rather own property.
The combination of AI and predictive analytics, a type of advanced analytics, allows organizations to take into account different factors from different disciplines to gain multi-disciplinary visibility into business problems, so that precise, fact-based and weighted solutions can be uncovered.
In even simpler terms, if advanced analytics can be used to see what’s not working, then machine learning can be used to uncover solutions that would work.
Analytics Solutions to Gain Contextual Intelligence Across the Supply Chain
It’s also possible to connect the supply chain with the stock market. For instance, production of corn may have been on the rise, but demand may have been unusually higher than any of the previous years. Perhaps a new FDA report, citing benefits of a nutrient found in corn, has received positive reviews, leading to a demand surge. It is now possible to correlate these findings with commodity options traded in the stock market thanks to AI, which given its dynamic nature is far more accommodating of incoming real-time data as compared to advanced analytics. Additionally, because of its feedback loop mechanism, AI can continuously revise its understanding of interconnected variables, which an advanced analytics solution might have previously missed.
This is another example of how an analytics model can be extended to uncover new patterns between seemingly disjointed variables with the help of AI. It has been established in many organizations across different industries that significant investments cannot be based on ‘gut check’ alone. AI findings can be used to revise existing advanced analytics models or to create new models, altogether. Organizations can also use deep learning models if they have technical know-how (such as a data scientist with domain knowledge) and vast troves of quality structured and unstructured data. However, success of any advanced analytics solution, complimented with or without AI, depends on these building blocks:
- The data scientist, Chief Analytics Officer or Chief Data Officer must have domain knowledge. Stoichiometry, Bayesian, Decision Tree, Random Forest, are just terms if they don’t actually solve an industry problem;
- IT and DevOps must understand integration A model is only considered to be deployed successfully if it is capable of interacting well with other systems;
- The performance of models should be continually monitored to see if they can be improved or have been rendered inefficient in the face of new data; or
- Analytics relies on the application of statistics, computer programming and operations Yet another reminder that for a model to be accepted, it needs to work in real world and tie with other routine operations. It can’t just be a successful science lab experiment.
Overall, advanced analytics is anything but obsolete and can serve as a foundational building block for successful AI models, whose findings in turn, can either be used to revise existing analytics models or craft entirely new algorithms.
About the author: Ankita Tyagi is the founder and principal analyst at FSInnovate. Tyagi was an analyst at State Street Bank and at the market research firm Aberdeen Group. Since 2017, Ankita has been tracking the progress of advanced technology, including AI and IoT, in general and specifically across Financial Services. She also explores the role of cloud computing, network, infrastructure, security, ethics, data laws, etc. in IoT and AI development.
Related Items:
Most Data Science Projects Fail, But Yours Doesn’t Have To
Do You Need a Chief Data Scientist?
The Secret to Data and Analytics Success Is…People