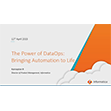
Data Science Needs to Grow Up, Domino Says
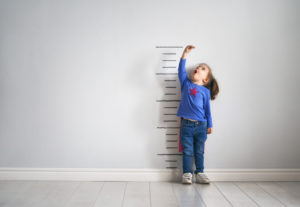
(Yuganov Konstantin/Shutterstock)
Nearly every American executive is leaning on data science to gain a competitive edge and boost profit, according to a newly released survey by Domino Data Lab. But flaws in the people, processes, and tools–not to mention a focus on “splashy” projects that lack substance–are holding back those data science dreams, the survey found.
Domino’s new study, dubbed the “Data Science Needs to Grow Up: The 2021 Domino Data Lab Maturity Index,” found that 71% of the 300 data execs at large companies it surveyed are counting on data science to drive revenue growth. In fact, 25% of the execs expect double-digit growth.
Despite the lofty ambitions, the companies are simply not making the investments necessary to achieve those results. According to the survey, a full 82% of execs admit that their company “makes splashy investments that have short-term payoffs.” Nearly half of the survey group (46%) say this happens “often or all the time.”
That isn’t going to cut it, says Nick Elprin, the CEO and co-founder at Domino Data Lab, the San Francisco-based provider of a data science platform and MLOps tools.
“We found that while executives have enormous expectations for revenue growth from their investments in data science, they are not making investments in the right places to truly unleash the power of data science,” Elprin stated in a press release. “To properly scale data science, companies need to invest in cohesive, sustainable processes to develop, deploy, monitor, and manage models at scale.”
Not surprisingly, Domino found correlations in the data that suggest certain attributes could be more predictive of big data success. For example, companies that exhibit a high maturity level were significantly more likely to treat data science as a “first-class discipline,” similar to finance or marketing, compared to their less mature brethren. Companies with a lower maturity level were more likely to treat data science as a support mechanism for disciplines like finance or marketing, the survey found.
Domino also published its Maturity Index for the first time within this report. The index shows how low-, medium-, and high-maturity data science organizations correspond with various attributes and aspects of the data science workflow, including the structure of the data science processes, the discoverability of assets, the speed and agility of the analytics, the breadth and depth of the data science impact, and organizational cohesion.
Not surprisingly, 50% of the high maturity companies report that their data science activites have a “great deal” of impact on their sales and revenues, the report found. By comparison, only 14% of companies with a low maturity data science organization say the data science projects have had a large impact.
The study also looked at the rate of abandonment of models, as well as the rate at which they are changed or improved. Nearly a quarter of models (23%) across all companies surveyed are not improved, while the percentage rises to 47% of models at low maturity companies.
Neglecting models can be disastrous to a company’s health, Domino warned. The survey found that 82% of executives are concerned about a major revenue loss or damage to brand reputation as a result of the failing models, while 46% worried about faulty models leading to wrong decisions and 45% expressed fear that they would adversely impact staffing and compensation decisions.
People are the key ingredient in many technological endeavors, and that applies to data science too. In Domino’s survey, a lack of data skills among employees was cited by 48% of executives as a top obstacle to achieving success with data science. That was followed by 39% who cited inconsistent standards and processes, 37% who cited outdated or inadequate tools, 34% who cited lack of buy-in from company leadership, and 34% who cited lack of data infrastructure and architecture.
“These obstacles are evidence that doing data science is hard, and progress requires a level-headed assessment of an organization’s “data science maturity” and associated resource needs for achieving the successful creation, deployment, and maintenance of production models at scale,” the company wrote in a blog post today.
Domino Data Lab commissioned Wakefield Research to prepare the report. You can download a copy of it here.
Related Items:
Are We Asking Too Much from Citizen Data Scientists?
On the Origin of Business Insight in a Data-Rich World
Data Science Success All About the Models, Domino Says