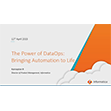
Bridging Intent with Action: The Ethical Journey of AI Democratization
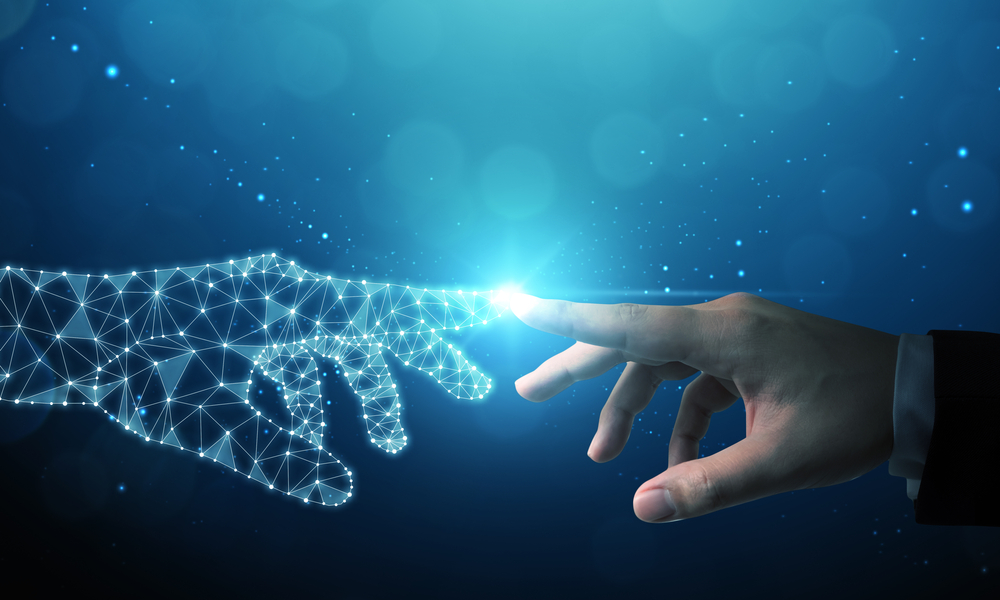
(Monster Ztudio/Shutterstock)
Artificial Intelligence (AI) is undergoing a profound transformation, presenting immense opportunities for businesses of all sizes. Generative AI has replaced traditional ML and AI as the hot topic in boardrooms. However, a recent Boston Consulting Group (BCG) study shows that more than half of the executives surveyed need help understanding GenAI. They are actively discouraging its use, while a further 37% indicate they are in a state of experimentation but have no policies or controls in place. In the following article, I will delve into the widespread accessibility of AI, analyze the associated obstacles and advantages, and learn about strategies for organizations to adapt to this ever-evolving field.
Companies should align governance and responsible AI practices with tangible business outcomes and risk management. Demonstrating how adherence to these guidelines can benefit the organization ethically and regarding bottom-line results helps garner stakeholder support and commitment at all levels.
Differentiating AI: Traditional vs. Generative AI
Distinguishing between traditional AI and generative AI is crucial for grasping the full scope of AI democratization. Traditional AI, which has existed for decades, provided a technique to analyze vast amounts of data to define a score or a pattern based on the learnings from the data. But the answers are always predictable – i.e., if the same question is asked ten times, the answer would remain the same. Creating the prediction or score often demands a specialized team of data scientists and experts to build and deploy models, making this less accessible to a broader audience within organizations.
Generative AI, on the other hand, represents a paradigm shift. It encompasses technologies like large language models that can create content in a human-like fashion based on the massive amounts of data used to train these models. In addition to the system being able to create new content (text, images, video, audio, etc.), it will constantly learn and evolve to the point that responses are no longer predictable or deterministic but will keep changing. This shift democratizes AI by making it accessible to a broader range of users, regardless of their specialized skill sets.
Balancing the Challenges and Risks of Rapid AI Adoption
Generative AI introduces unique challenges, mainly when relying on prepackaged solutions. The concept of explainability in AI presents a significant challenge, particularly in traditional AI systems where outcomes are often presented as simple probability scores like “0.81” or “loan denied.” Deciphering the reasoning behind such scores typically requires specialized knowledge, raising questions about fairness, potential biases stemming from profiling, and other factors influencing the outcome.
When discussing explainability within the realm of GenAI, it’s crucial to examine the sources behind the explanations provided, particularly in the case of open LLMs such as OpenAI or Llama. These models are trained on vast amounts of internet data and GitHub repositories, raising concerns about the origin and accuracy of responses and potential legal risks related to copyright infringement. Moreover, fine-tuning embeddings often feed into vector databases, enriching them with qualitative information. The question of data provenance remains pertinent. However, if someone were to input their support tickets into the system, they would have a clearer understanding of the data’s origins.
While the democratization of GenAI presents immense value, it also introduces specific challenges and risks. The rapid adoption of GenAI can lead to concerns related to data breaches, security vulnerabilities, and governance issues. Organizations must strike a delicate balance between capitalizing on the benefits of GenAI and ensuring data privacy, security, and regulatory compliance.
It’s critical to clearly understand the risks, practical solutions, and best practices for implementing responsible GenAI. When employees understand the potential risks and the strategies to navigate said risks, they are more likely to embrace responsible GenAI practices and are better positioned to navigate challenges effectively. Taking a balanced approach fosters a culture of responsible AI adoption.
Responsible AI: Bridging the Gap Between Intent and Action
Organizations are increasingly establishing responsible GenAI charters and review processes to address the challenges of GenAI adoption. These charters guide ethical GenAI use and outline the organization’s commitment to responsible GenAI practices. However, the critical challenge is bridging the gap between intent and action when implementing these charters. Organizations must move beyond principles to concrete actions that ensure GenAI is used responsibly throughout its lifecycle.
To maximize AI’s benefits, organizations should encourage different teams to experiment and develop their GenAI apps and use cases while providing prescriptive guidance on the necessary controls to adhere to and which tools to use. This approach ensures flexibility and adaptability within the organization, allowing teams to tailor solutions to their specific needs and objectives.
Building a Framework That Opens Doors to Transparency
AI is a dynamic field characterized by constant innovation and evolution. As a result, frameworks for responsible AI must be agile and capable of incorporating new learnings and updates. Organizations should adopt a forward-looking approach to responsible AI, acknowledging that the landscape will continue to evolve. As transparency becomes a central theme in AI governance, emerging regulations driven by organizations like the White House may compel AI providers to disclose more information about their AI systems, data sources, and decision-making processes.
Effective monitoring and auditing of AI systems are essential to responsible AI practices. Organizations should establish checkpoints and standards to ensure compliance with responsible AI principles. Regular inspections, conducted at intervals such as monthly or quarterly, help maintain the integrity of AI systems and ensure they align with ethical guidelines.
Privacy vs. AI: Evolving Concerns
Privacy concerns aren’t new and have existed for some time now. However, the fear and understanding of AI’s power have grown in recent years, contributing to its popularity across industries. AI is now receiving increased attention from regulators at both the federal and state levels. Growing concerns about AI’s impact on society and individuals are leading to heightened scrutiny and calls for regulation.
Enterprises should embrace privacy and security as enablers rather than viewing them as obstacles to AI adoption. Teams should actively seek ways to build trust and privacy into their AI solutions while simultaneously achieving their business goals. Striking the right balance between privacy and AI innovation is essential.
Democratization of AI: Accessibility and Productivity
Generative AI’s democratization is a game-changer. It empowers organizations to create productivity-enhancing solutions without requiring extensive data science teams. For instance, sales teams can now harness the power of AI tools like chatbots and proposal generators to streamline their operations and processes. This newfound accessibility empowers teams to be more efficient and creative in their tasks, ultimately driving better results.
Moving Toward Federal-Level Regulation and Government Intervention
Generative AI regulatory frameworks will move beyond the state level towards federal and country-level standards. Various working groups and organizations are actively discussing and developing standards for AI systems. Federal-level regulation could provide a unified framework for responsible AI practices, streamlining governance efforts.
Given the broad implications of AI decision-making, there is a growing expectation of government intervention to ensure responsible and transparent AI practices. Governments may assume a more active role in shaping AI governance to safeguard the interests of society as a whole.
In conclusion, the democratization of AI signifies a profound shift in the technological landscape. Organizations can harness AI’s potential for enhanced productivity and innovation while adhering to responsible AI practices that protect privacy, ensure security, and uphold ethical principles. Startups, in particular, are poised to play a vital role in shaping the responsible AI landscape. As the AI field evolves, responsible governance, transparency, and a commitment to ethical AI use will ensure a brighter and more equitable future for all.
About the author: Balaji Ganesan is CEO and co-founder of Privacera. Before Privacera, Balaji and Privacera co-founder Don Bosco Durai also founded XA Secure. XA Secure was acquired by Hortonworks, who contributed the product to the Apache Software Foundation and rebranded as Apache Ranger. Apache Ranger is now deployed in thousands of companies around the world, managing petabytes of data in Hadoop environments. Privacera’s product is built on the foundation of Apache Ranger and provides a single pane of glass for securing sensitive data across on-prem and multiple cloud services such as AWS, Azure, Databricks, GCP, Snowflake, and Starburst and more.
Related Items:
GenAI Doesn’t Need Bigger LLMs. It Needs Better Data
Top 10 Challenges to GenAI Success
Privacera Report Shows That 96% of Businesses are Pursuing Generative AI for Competitive Edge