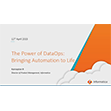
Project Co-led at LLNL Looks to Improve Visualization of Largescale Datasets
Oct. 28, 2022 — Lawrence Livermore National Laboratory researchers are starting work on a three-year project aimed at improving methods for visual analysis of large heterogeneous data sets as part of a recent Department of Energy funding opportunity.
The joint project, entitled “Neural Field Processing for Visual Analysis,” will be led at LLNL by co-principal investigator (PI) Andrew Gillette. Gillette is joined by lead PI Matthew Berger at Vanderbilt University and co-PI Joshua Levine at the University of Arizona.
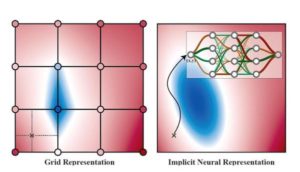
The processing of grid-based data (left) relies on choices made for grid connectivity and interpolation, notions that are absent within implicit neural representations (INRs, right). A newly funded project co-led by Lawrence Livermore National Laboratory researchers will enable visual analysis processing methods on INRs directly, without a traditional grid representation. Credit: Andrew Gillette
While visualization is essential to understanding results of numerical simulations, modern data sets can be large in size and heterogeneous in type, making direct processing computationally challenging, Gillette said.
The newly funded project will explore methods for processing “implicit neural representations” (INRs) — datasets that incorporate coordinate-based neural networks to represent scientific data sets efficiently and compactly. Currently, traditional processing algorithms and visual analysis techniques cannot be applied to INRs directly, Gillette explained.
“It’s an honor to have been selected to carry out this research for the DOE,” Gillette said. “Fast and accurate visualization is essential for a wide variety of activities underway at DOE laboratories; my goal over the next three years is to partner closely with application domain specialists and demonstrate how advances in visualization methodologies can directly benefit scientific inquiry.”
Gillette, who took over the PI role from former LLNL computer scientist Harsh Bhatia, said the project will encode key features of large data sets via emerging INR techniques. In addition, it will provide methods for rapidly extracting and interacting with data set features using only the compactly represented INR as a data surrogate, instead of maintaining access to the complete data set. As an example, the methods could be applied to visual interfaces for computational fluid dynamics simulations, which contain important fine-scale geometric and topological features in a large 3D region.
The new project was one of five awarded funding in September under the DOE’s “Data Visualization for Scientific Discovery, Decision-Making, and Communication” Funding Opportunity Announcement (FOA). The goal of the FOA is to advance visualization techniques to address challenges from the rapid expansion of data generation and complexity of data types produced by scientific experiments and simulations performed on modern supercomputers.
The advances will address emerging visualization technologies, enable better interdisciplinary collaboration and enhance communication across domains, according to DOE. Total funding for scientific data visualization research was $12.5 million.
Source: LLNL